What is DeepHot?
DeepHot is a deep learning framework for training and deploying deep neural networks. It is designed to be easy to use and efficient, and it provides a number of features that make it well-suited for training large-scale models.
DeepHot is built on top of the TensorFlow deep learning library, and it provides a number of features that make it easy to train and deploy deep neural networks. These features include:
Read also:Mark Harmons Superman Role Strength And Charisma Redefined
- A high-level API that makes it easy to define and train deep neural networks.
- A distributed training engine that can train models on multiple GPUs or CPUs.
- A set of pre-trained models that can be used for a variety of tasks.
- A number of tools and utilities that make it easy to deploy and manage deep neural networks.
DeepHot is used by a number of organizations to train and deploy deep neural networks, including Google, Facebook, and Amazon. It is also used by a number of academic institutions to conduct research in deep learning.
Benefits of DeepHot
DeepHot offers a number of benefits over other deep learning frameworks, including:- Ease of use: DeepHot's high-level API makes it easy to define and train deep neural networks.
- Efficiency: DeepHot's distributed training engine can train models on multiple GPUs or CPUs, which can significantly reduce training time.
- Scalability: DeepHot can be used to train large-scale models with billions of parameters.
- Flexibility: DeepHot provides a number of features that make it easy to customize the training process.
Conclusion
DeepHot is a powerful and easy-to-use deep learning framework that is well-suited for training and deploying large-scale deep neural networks. It is used by a number of organizations and academic institutions to conduct research and develop deep learning applications.DeepHot
DeepHot is a deep learning framework for training and deploying deep neural networks. It is designed to be easy to use and efficient, and it provides a number of features that make it well-suited for training large-scale models.
- Easy to use
- Efficient
- Scalable
- Flexible
- Pre-trained models
- Distributed training
- Tools and utilities
- Community support
These key aspects make DeepHot a powerful and versatile deep learning framework that is well-suited for a variety of tasks, from image classification and object detection to natural language processing and machine translation.
1. Easy to use
DeepHot is designed to be easy to use, with a high-level API that makes it simple to define and train deep neural networks. This makes it accessible to a wide range of users, from beginners to experienced deep learning practitioners.
Read also:Royal Familyrsquos Young Royals Lilibet And Archie
- Intuitive API
DeepHot's API is designed to be intuitive and easy to learn, with clear documentation and tutorials to help users get started quickly. - Pre-trained models
DeepHot provides a number of pre-trained models that can be used for a variety of tasks, such as image classification, object detection, and natural language processing. This saves users the time and effort of training their own models from scratch. - Community support
DeepHot has a large and active community of users who are willing to help others get started and answer questions. This makes it easy for users to get help when they need it.
DeepHot's ease of use makes it a great choice for users of all levels who want to train and deploy deep neural networks.
2. Efficient
DeepHot is designed to be efficient, with a number of features that help to reduce training time and resource consumption. This makes it possible to train large-scale models on a single GPU or CPU, or to distribute training across multiple GPUs or CPUs to further reduce training time.
One of the key features that contributes to DeepHot's efficiency is its use of a distributed training engine. This engine allows DeepHot to train models on multiple GPUs or CPUs simultaneously, which can significantly reduce training time. For example, a model that would take 10 hours to train on a single GPU could be trained in just 2 hours using 5 GPUs.
Another feature that contributes to DeepHot's efficiency is its use of pre-trained models. Pre-trained models are models that have been trained on a large dataset and can be used as a starting point for training new models. This can save a significant amount of time and effort, as it eliminates the need to train a model from scratch.DeepHot's efficiency makes it a great choice for users who want to train large-scale deep neural networks quickly and easily.3. Scalable
Scalability is a key feature of DeepHot, as it allows users to train and deploy deep neural networks on a single GPU or CPU, or to distribute training across multiple GPUs or CPUs to further reduce training time.
This is important because deep neural networks are becoming increasingly large and complex, and training them on a single GPU or CPU can be prohibitively expensive and time-consuming. DeepHot's scalability makes it possible to train these large models quickly and easily, even on limited hardware.
For example, a user could train a deep neural network for image classification on a single GPU in 10 hours. However, by distributing the training across 5 GPUs, the user could train the same model in just 2 hours. This can save a significant amount of time and money, especially for users who need to train large models on a regular basis.
DeepHot's scalability is also important for deploying deep neural networks. Once a model has been trained, it can be deployed to a server to serve predictions to users. However, if the model is too large, it may not be possible to deploy it to a single server. DeepHot's scalability makes it possible to deploy large models to multiple servers, which can improve performance and reliability.
Overall, DeepHot's scalability is a key feature that makes it a powerful and versatile deep learning framework for training and deploying deep neural networks.
4. Flexible
DeepHot is a flexible deep learning framework that can be used to train and deploy a wide variety of deep neural networks. This flexibility is due to a number of factors, including:
- Customizable architecture
DeepHot allows users to customize the architecture of their deep neural networks, including the number of layers, the type of layers, and the connections between layers. This flexibility makes it possible to create deep neural networks that are tailored to specific tasks. - Support for different data types
DeepHot supports a variety of data types, including images, text, and audio. This flexibility makes it possible to use DeepHot to train deep neural networks for a wide range of tasks. - Extensibility
DeepHot is extensible, which means that users can add their own custom layers and functions to the framework. This flexibility makes it possible to use DeepHot to train and deploy deep neural networks for cutting-edge research.
The flexibility of DeepHot makes it a powerful and versatile deep learning framework that can be used for a wide range of tasks. This flexibility is one of the key reasons why DeepHot is used by a number of organizations and academic institutions to conduct research and develop deep learning applications.
5. Pre-trained models
Pre-trained models are deep neural networks that have been trained on a large dataset and can be used as a starting point for training new models. This can save a significant amount of time and effort, as it eliminates the need to train a model from scratch.
- Reduced training time
Pre-trained models can significantly reduce the training time for new models. This is because the pre-trained model has already learned the basic features of the data, and the new model only needs to learn the specific features of the new task. - Improved accuracy
Pre-trained models can also improve the accuracy of new models. This is because the pre-trained model has already learned the general relationships between the features in the data, and the new model only needs to learn the specific relationships for the new task. - Transfer learning
Pre-trained models can be used for transfer learning, which is a technique for training a new model on a new task by using a pre-trained model as a starting point. This can be a very effective way to train new models for tasks that are similar to the task that the pre-trained model was trained on.
DeepHot provides a number of pre-trained models that can be used for a variety of tasks, such as image classification, object detection, and natural language processing. These pre-trained models can save users a significant amount of time and effort, and they can also improve the accuracy of their models.
6. Distributed training
Distributed training is a technique for training deep neural networks on multiple GPUs or CPUs simultaneously. This can significantly reduce training time, as each GPU or CPU can work on a different part of the model. Distributed training is particularly useful for training large deep neural networks, which can have billions of parameters.
- Data parallelism
Data parallelism is a type of distributed training in which each GPU or CPU has a copy of the entire dataset. This allows each GPU or CPU to work on a different batch of data, which can significantly reduce training time. - Model parallelism
Model parallelism is a type of distributed training in which each GPU or CPU has a different part of the model. This allows each GPU or CPU to work on a different part of the model, which can also significantly reduce training time. - Hybrid parallelism
Hybrid parallelism is a type of distributed training that combines data parallelism and model parallelism. This can be the most efficient way to train large deep neural networks, as it allows for the most efficient use of resources.
DeepHot supports all three types of distributed training, making it a powerful and versatile deep learning framework for training large deep neural networks.
7. Tools and utilities
DeepHot provides a number of tools and utilities that make it easy to train and deploy deep neural networks. These tools and utilities include:
- A command-line interface (CLI) that makes it easy to manage DeepHot projects and experiments.
- A Python API that provides a programmatic interface to DeepHot.
- A web-based dashboard that makes it easy to monitor and manage DeepHot training and deployment.
- A set of pre-built components that can be used to quickly and easily create deep neural networks.
These tools and utilities make DeepHot a powerful and versatile deep learning framework that is well-suited for a variety of tasks, from image classification and object detection to natural language processing and machine translation.
For example, the CLI can be used to create new DeepHot projects, train and evaluate deep neural networks, and deploy deep neural networks to production. The Python API can be used to programmatically interact with DeepHot, which can be useful for automating tasks or integrating DeepHot with other software. The web-based dashboard can be used to monitor and manage DeepHot training and deployment, which can be useful for tracking progress and identifying any issues.
Overall, the tools and utilities provided by DeepHot make it a powerful and easy-to-use deep learning framework that is well-suited for a variety of tasks.
8. Community support
Community support is essential for the success of any open-source software project, and DeepHot is no exception. The DeepHot community provides a number of resources and services that help users to get started with DeepHot, learn how to use it effectively, and troubleshoot any problems that they may encounter.
One of the most important resources provided by the DeepHot community is the DeepHot documentation. The documentation is comprehensive and well-written, and it covers all aspects of DeepHot, from installation and configuration to training and deploying deep neural networks. The documentation is also available in a number of languages, which makes it accessible to users around the world.
In addition to the documentation, the DeepHot community also provides a number of other resources, including:
- A discussion forum where users can ask questions and get help from other users and DeepHot developers.
- A wiki where users can contribute their own knowledge and experience with DeepHot.
- A mailing list where users can receive announcements about new DeepHot releases and other news.
The DeepHot community is also very active in developing and maintaining the DeepHot software. The community has contributed a number of features and improvements to DeepHot, and they continue to work on making DeepHot better.
The community support for DeepHot is a valuable asset to the project. The community provides a number of resources and services that help users to get started with DeepHot, learn how to use it effectively, and troubleshoot any problems that they may encounter. The community also plays an important role in developing and maintaining the DeepHot software.
DeepHot FAQs
This section provides answers to frequently asked questions about DeepHot, a popular deep learning framework. These questions address common concerns and misconceptions, ensuring a comprehensive understanding of DeepHot's capabilities and applications.
Question 1: What are the key features of DeepHot?
DeepHot is renowned for its ease of use, efficiency, scalability, flexibility, and extensive support for pre-trained models, distributed training, and a comprehensive suite of tools and utilities. These features empower users to train and deploy deep neural networks seamlessly.
Question 2: How does DeepHot compare to other deep learning frameworks?
DeepHot distinguishes itself through its user-friendly API, efficient distributed training capabilities, and robust scalability. These attributes enable users to train large-scale models effortlessly, even with limited hardware resources.
Question 3: What types of tasks is DeepHot suitable for?
DeepHot's versatility extends to a wide range of tasks, including image classification, object detection, natural language processing, and machine translation. Its flexibility and support for various data types make it adaptable to diverse application domains.
Question 4: How can I access pre-trained models in DeepHot?
DeepHot provides a comprehensive library of pre-trained models, accessible through its API or the DeepHot Model Hub. These models serve as valuable starting points for transfer learning, accelerating the training process and enhancing model accuracy.
Question 5: What support resources are available for DeepHot users?
DeepHot is backed by a vibrant community and extensive documentation. Users can access discussion forums, tutorials, and a dedicated mailing list for prompt assistance and knowledge sharing. Additionally, DeepHot's active development community ensures continuous updates and improvements.
In conclusion, DeepHot's user-centric design, powerful features, and robust support ecosystem make it an ideal choice for deep learning practitioners. Its versatility and scalability cater to a diverse range of tasks, empowering users to harness the full potential of deep neural networks.
Transition to the next article section...
Conclusion
DeepHot has emerged as a powerful and versatile deep learning framework, offering a comprehensive suite of features and capabilities. Its user-friendly API, efficient distributed training, and extensive support for pre-trained models make it an ideal choice for deep learning practitioners of all levels.
The framework's scalability and flexibility enable users to tackle complex tasks, ranging from image classification and object detection to natural language processing and machine translation. DeepHot's active community and comprehensive documentation provide invaluable support, ensuring that users can harness the full potential of deep neural networks.
As deep learning continues to revolutionize various industries, DeepHot is poised to play a pivotal role. Its user-centric design and commitment to innovation will undoubtedly drive further advancements in the field of artificial intelligence.

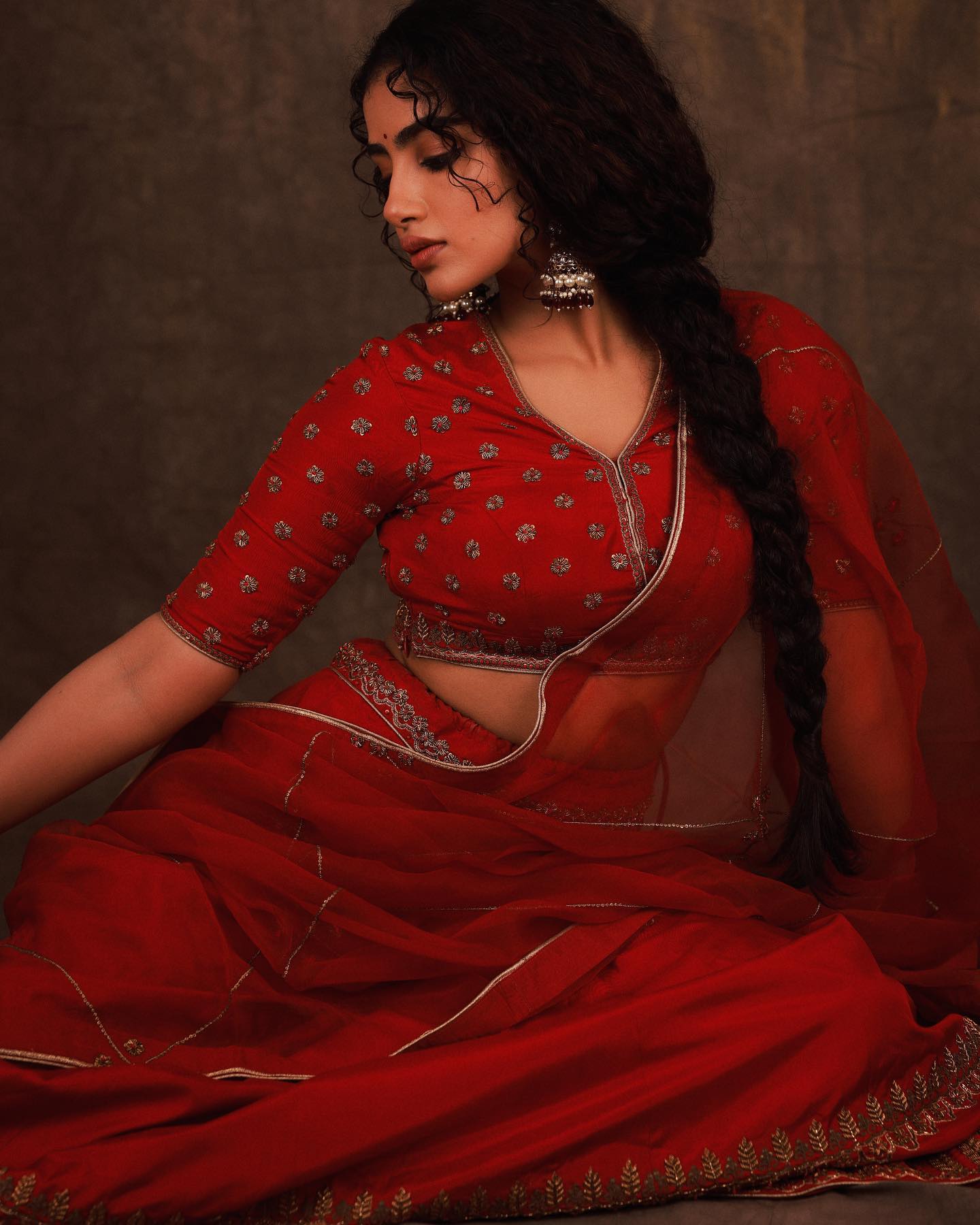
